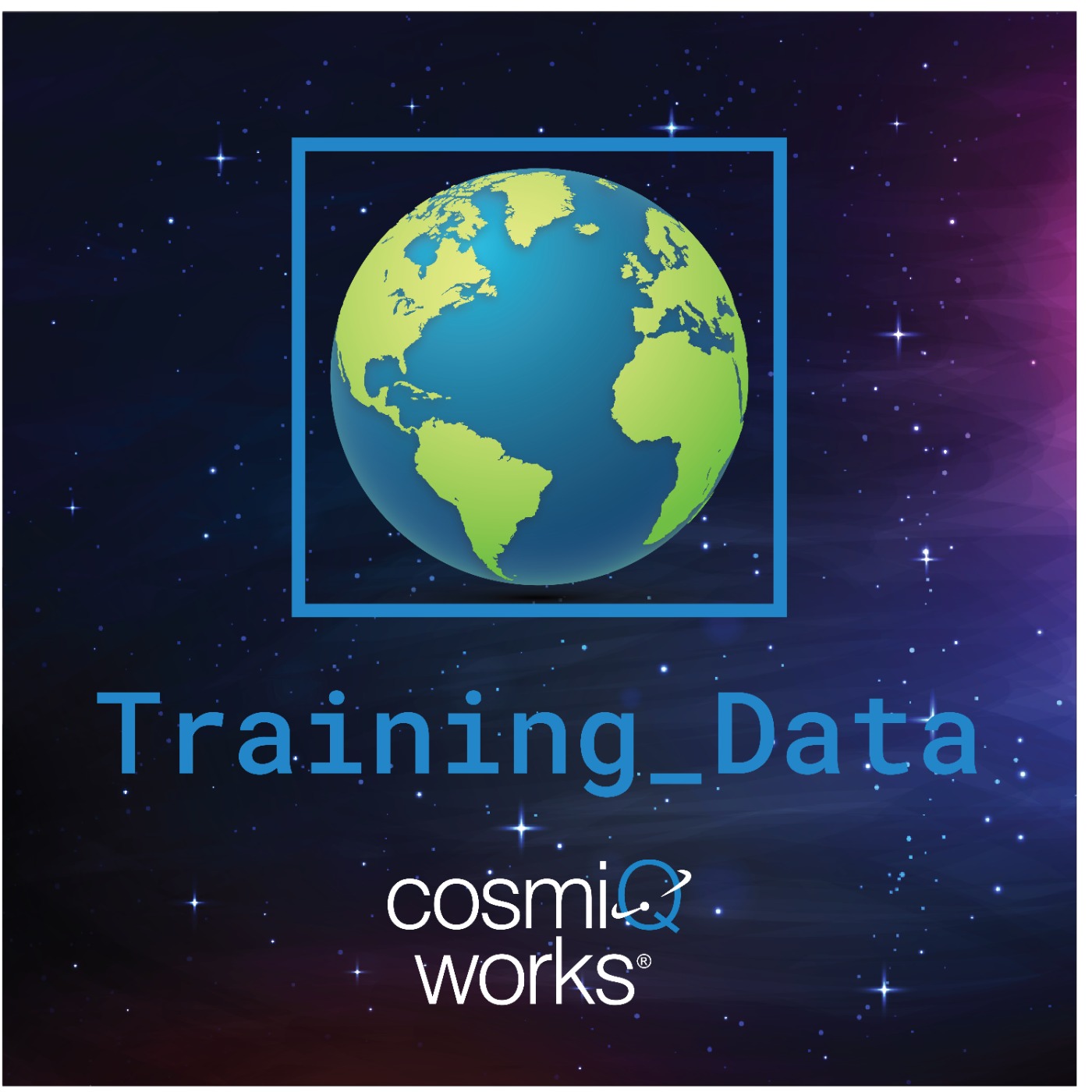
Episode description
How much labeled data does a machine learning model need in order to achieve sufficient performance? Most analyses simply use all available data and focus on model architecture, with scant attention given to whether the dataset size is appropriate for the task and architecture’s complexity. But is that the right answer?
In this episode, IQT CosmiQ Works’ Ryan Lewis, Daniel Hogan, and Adam Van Etten dive into the importance of deep quantitative analysis and the impact of training data size on model performances. Learn how they used CosmiQ’s Machine Learning Robustness Study to help address dependence on geography and dataset size at the leading edge of geospatial machine learning.
Learn more about CosmiQ at www.cosmiqworks.org.